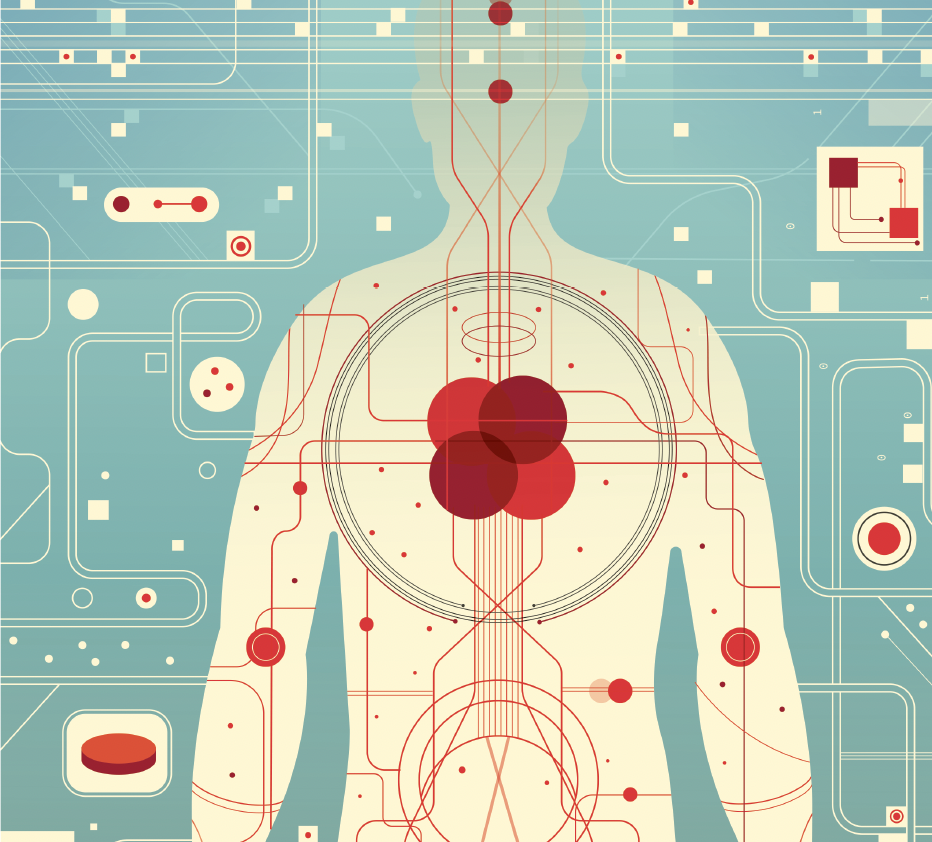
Precision EM: Digital Behavioral Interventions
Brian Suffoletto, MD is designing and testing digital behavioral interventions to expand care options before and after a patient visits the emergency department.
Using Digital Devices to Expand Care Beyond the Emergency Department
As an associate professor of emergency medicine, Brian Suffoletto, MD sees emergency department (ED) patient encounters as opportunities to identify individuals with specific risks and connect them to effective interventions that help them adopt behavioral changes after they leave the ED.
Suffoletto, associate professor of emergency medicine, is designing and testing digital behavioral interventions (DBIs) across a range of conditions. His work centers on improving the health of older adolescents and young adults, given their increased relative risks for certain health issues (e.g. alcohol and mental health issues) and the ubiquity of smartphone ownership, as well as their use of the ED as a surrogate for primary care.
Suffoletto is leading multi-site clinical trials testing the use of DBIs with young adults to:
improve seatbelt use, lower distracted driving, and reduce drinking and driving
determine optimal strategies to reduce hazardous alcohol consumption.
improve mental health care for depression and mental health self-management in the transition to college.
Human-computer interaction (HCI)
Although an app might seem to be most effective in engaging individuals in behavior change, 50% of app users will stop using them after 30 days. In multiple studies, Suffoletto has found that conducting automated conversations through text messaging results in higher impact and sustained engagement.
Acknowledging and rewarding user input is critical to engagement. For youth with depression, Suffoletto developed a DBI to monitor mood that provides feedback on trends and contextual triggers each time an individual enters data. “Simple insights that are neither intuitive nor obvious,” he notes, “but that incentivize the user to continue to engage.”
For DBIs that require lengthy engagement, Suffoletto includes the voluntary option to take a two-week break. In pilot research, when allowing these vacations, he and his team found engagement rates sustained at over 80% after one year.
Context-aware computing
Most DBIs require users to take time to input data. Context-aware computing uses data collected passively across all devices – phone, watch, etc. – throughout an individual’s daily life to infer certain conditions such as depression, craving, sickness, and more.
Suffoletto recently used context-aware computing to develop an algorithm that employs accelerometry from a phone in a pocket to detect gait impairment due to drug or alcohol use. The potential applications are wide-ranging; for instance, the same algorithm could also be used to identify fall risk in elderly patients.
“Changing certain behaviors once they begin can be difficult. The challenge is finding the best time to intervene prior to the behavior but soon enough to be contextually relevant,” he explains. “If we can use passively-collected data to infer certain states, then we can intervene just in time.”
The specific strategies used in Suffoletto’s DBIs are informed by psychological theories of behavior change. For example, Suffoletto found that young adults with hazardous drinking will commit to goals to reduce drinking if the goal is closer to their recent drinking amounts, and an algorithm that steps them down gradually over time is much more effective. Suffoletto has also found that a goal not met can be negatively self-reinforcing. Suffoletto hopes to develop automated tools to reduce goal limit violation effects on subsequent goal-striving.